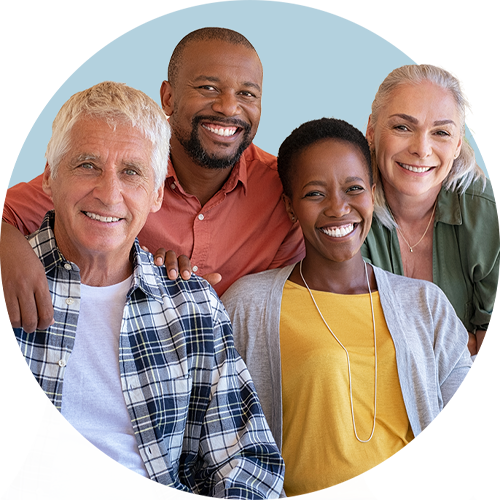
Artificial intelligence and machine learning can drive significant improvements in healthcare but concerns around their effectiveness are reasonable. How can the industry responsibly use these technologies to reach the right patients with meaningful content?
Artificial intelligence. Natural language processing. Machine learning.
These terms can stir up heated conversations and debates, raising questions about the societal, ethical and economic implications of technology—especially in the healthcare industry. Concerns about artificial intelligence (AI) and machine learning (ML) are reasonable and warranted, as these systems can be prone to errors, particularly if they are trained on incomplete data.
“[These technologies] aren’t a panacea and don’t magically produce better quality or efficiency in healthcare,” says Dr. Luke Goetzke, Director of Data Science, Phreesia Life Sciences. “Depending on how these tools are used, they can be biased or produce results that are simply wrong.”
However, when implemented responsibly, these technologies can drive significant improvements and be extremely effective at solving certain types of problems, especially when human experts are involved to help avoid potential pitfalls, Dr. Goetzke says. At Phreesia, we leverage AI, ML and natural language processing (NLP) in responsible ways to better understand patient populations and help life science brands and government organizations reach the right patients with truly meaningful content. Here’s how.
1. We use NLP to synthesize and interpret different ways of answering the same questions
Phreesia works with healthcare organizations across the United States—and they all collect data in different ways. In 2023, Phreesia managed more than 150 million patient visits, which means a large amount of rich, unstructured patient data. Synthesizing and interpreting it safely and effectively calls for advanced methods that prioritize security.
For example, patient-supplied answers that are gathered during the intake process can help identify what content is relevant for a unique person. Answers are sometimes selected from a supplied list of options and are sometimes free text. NLP techniques help address the many ways people can express the same idea and how words or phrases can have different meanings in different contexts, Dr. Goetzke says.
Consider, for example, medical acronyms and abbreviations: In one context, the letters “CAS” might mean carotid artery stenosis, but in others, it might mean cerebral arteriosclerosis. Or those letters could appear inside a different yet common word, like casualty. “Trying to exhaustively list all the ways that snippets of text can be presented would be very challenging, if not impossible,” Dr. Goetzke says.
Phreesia uses NLP techniques to more clearly define abstract rules or models that can help identify the likely meaning of words in context—as well as filter out non-relevant answers. These techniques can help identify whether a word is a medication, symptom or name, and how these words are related to each other in a sentence. NLP tools can also help identify when the meaning of a sentence has been negated.
2. We use AI to make projections about expected future behavior
As a business, one challenge is anticipating what the patient population is going to look like in a year or even five years. For example, we might have data on how many patients are being treated for chronic kidney disease (CKD) today, but how many patients do we expect to be treated for CKD a year from now? These types of projections and forecasts can help us identify patterns in healthcare and population trends to better understand patients and better serve our clients.
To make these projections, we leverage a variety of time-series ML and AI methods, such as seasonal autoregressive models and other statistical techniques. These methods look for patterns in what we have observed in past years to extrapolate what might happen next year, Dr. Goetzke says.
3. We use AI to define relevant clinical audiences for campaigns
Our research shows that, when given the option, patients like content that is tailored to address their unique needs, and they welcome receiving that messaging at the point of care. But getting the right message to the right patient when they consent to see it requires an in-depth analysis of data, while also preserving privacy, and that is made possible through AI tools and technologies.
“I think this is an area where Phreesia is really on the cutting edge and using AI and ML in a smart way,” Dr. Goetzke says.
Phreesia’s Data Science team is actively developing knowledge graphs and large language models (LLMs) to accelerate the audience definition process, Dr. Goetzke says. A knowledge graph connects data points by linking relevant information—like symptoms, medications and diagnoses—to show how they are connected. The knowledge graphs that Phreesia’s team is developing are trained on third-party data from sources like the National Institutes of Health about medical concepts and the relationship between them, as well as Phreesia data. “The interface is like a library search tool on steroids,” Dr. Goetzke says.
Without this tool, a member of Phreesia’s Analytics and Insights team would be tasked with making those connections and accurately and reliably identifying the type of patient a client wants to reach. While doable, it’s a time-consuming process, Dr. Goetzke says.
“The biggest benefit that I think these tools can have right now is cutting out a lot of the leg work and saving time,” he says. “But this is also where we really leverage human-in-the-loop (HITL). While humans are not looking at patients’ unique data, an Analytics and Insights team member does review recommendations for audience definitions to validate them, add nuance and fine-tune the results.”
Additionally, Phreesia continually reevaluates and validates audience definitions and model outputs—a critical step in reducing bias and errors, Dr. Goetzke says. “The risk for AI and ML failing is higher when it is being applied to data it has never seen before,” he says. “That’s why we regularly reevaluate the performance of our models on ‘out of sample’ data. Sometimes we discover new cases or edge cases where we need to teach our models to behave differently. We leverage a combination of ML, AI and HITL for this type of evaluation as well, enabling us to do this efficiently at scale.”
In all use cases for AI tools and technologies, Phreesia keeps humans involved in the entire process to reduce errors and validate results. “I think real human intelligence is better than artificial intelligence, at least for now,” Dr. Goetzke says. “I’m excited about the time that we live in and the technology advances that we’ve made, but I think knowledge and wisdom are still coming from people.”
Learn how Phreesia can leverage AI, ML, NLP and HITL to help you reach the right patients when they are in a healthcare state of mind.
Glossary of Terms:
Artificial intelligence: Refers to a field of science that involves building machines and computers that can perform human-like tasks. AI learns new information and improves output through exposure to large amounts of data.
Machine learning: A type of artificial intelligence that allows machines and computers to learn and improve from experience—not from being explicitly programmed.
Natural language processing: A type of artificial intelligence that uses machine learning to understand, process and generate human language. It can be used to analyze the intent in a message, summarize documents and identify patterns.
Human-in-the-loop: When humans are involved in the development and training of AI and ML systems, this collaboration is known as “human-in-the-loop.”